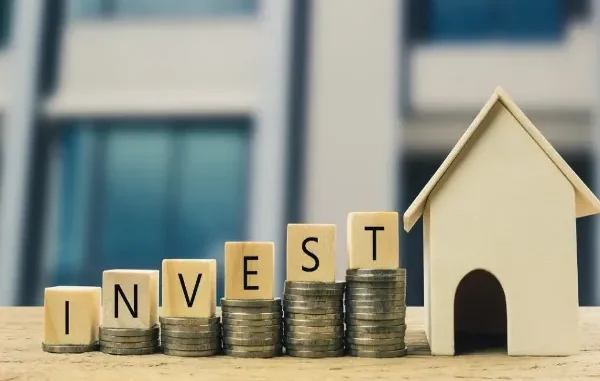
AI signals are revolutionizing trading, providing data-driven insights that help traders stay ahead. But how do traders measure their effectiveness? Key metrics like accuracy, profitability, and adaptability come into play. Evaluating AI performance requires a blend of technology and judgment to ensure reliable outcomes. Let’s explore how traders assess the value of these advanced tools. Have you explored how Gas +900 Evex connects traders with trusted educational firms to better understand the potential of AI signals in trading? It’s an intriguing way to bridge the gap between tools and expertise.
Selecting The Right Key Performance Indicators (Kpis) For Ai Signals
Deciding on suitable KPIs can feel like juggling watermelons while keeping an eye on a stopwatch. A positive trend in 2023 shows that traders are pinpointing more effective markers for their AI-driven tactics. An eager observer might ask, “Why so many metrics?” The explanation often lies in the different goals set by each participant. Some focus on frequent small gains. Others lean on fewer trades but aim for higher returns.
Win Rate Versus Profit Factor
A high win rate might sound glorious. Yet profit factor measures net profit over net loss,
which pinpoints whether gains outweigh costs. It can stop a strategy that looks decent on paper but drains the account behind the scenes.
Sharpe Ratio, Sortino Ratio, And Beyond
Sharpe Ratio suits those who prize a steady journey over sudden leaps. Sortino Ratio zeroes in on downside risks, which offers a sharper view when volatile markets leave folks feeling like they’re riding a roller coaster. A glance at these numbers can spark a moment of truth. Is a strategy stable enough for large sums? Or is it riskier than a leap off a tall diving board?
Drawdown And Maximum Adverse Excursion
Temporary dips happen. Drawdown gauges the peak-to-valley difference. Maximum Adverse Excursion takes that idea and highlights the deepest loss a position faced before bouncing back. Anyone spotting a pattern of repeated deep dips might think about adjusting position sizes.
Questions arise. How often is analysis updated? Is there a plan for ongoing revisions? Keeping data fresh and double-checking results can be beneficial. Skilled advisors and thorough research remain priceless for these pursuits.
Advanced Methodologies For Evaluating Ai Performance
Some folks compare old-fashioned evaluation to peering through a straw. Tools have become sharper, and more data streams in than ever before. A quick query: Should a model remain static in a sea of shifting prices? Probably not.
Cross-Validation And Out-Of-Sample Testing
Cross-validation breaks historical data into multiple slices, then shuffles them around for thorough checks. It reduces guesswork about overfitting. Out-of-sample testing adds an extra layer of objectivity by reserving data the model never saw during training.
Rolling Window Analysis And Robustness Checks
Markets can shift faster than a magician’s sleight of hand. Rolling window analysis updates models at set intervals, which can show how they handle fresh conditions. Robustness checks help confirm consistent results across different timeframes. That might spark a thought: If markets pivot on a dime, does the model adapt quickly or does it stumble?
Bayesian Approaches To Performance Assessment
There is an approach that blends old-school probability with fresh data. Bayesian methods gradually update beliefs rather than discarding previous insights. Some who track data daily prefer this style, since partial updates can reduce abrupt swings in strategy. A friend once joked about reading a weather forecast and never glancing outside. Bayesian updates resemble that actual peek through the window each morning.
As always, any method can malfunction without professional guidance. Research groups and licensed professionals often have real-world views that algorithms might miss.
Interpreting Ai Insights With Explainable Ai (Xai)
Some folks joke about AI insights feeling like cryptic riddles. The challenge involves understanding how signals emerge, especially if large sums ride on every decision.
The Black Box Problem In Financial Algorithms
Opaque systems puzzle many. Complex layers can hide the cause behind a buy-or-sell alert. It can seem like trusting a fortune-teller who never reveals a method. No one wants to watch an account balance shift without a clue about the root cause.
Techniques To Derive Explainability
Developers often tinker with simpler models or post-hoc interpretability methods. Tools may rank key drivers of trade decisions. Some highlight data points that pushed signals in certain directions. Ever watched a detective show where the investigator reveals the hidden clue at the end? That sense of discovery can bring relief and accountability.
Balancing Accuracy And Explainability
Accuracy might skyrocket with deeper networks, yet interpretability can dip. Not every scenario demands perfect clarity, but a rough sense of logic can comfort risk managers. Here’s a single set of tips:
- Pick models that fit the complexity of the market.
- Double-check if insights resonate with known patterns.
- Confirm that daily adjustments match personal risk preferences.
Periodic reviews can prove worthwhile. A transparent process helps form trust, which matters when major funds hang in the balance. Feeling unsure? Consultation with seasoned experts and extra reading can calm nerves. Nobody wants confusion to guide critical investment moves.
Conclusion
The performance of AI signals is judged by their ability to deliver consistent and actionable insights. From predicting trends to enhancing trading efficiency, AI tools have become indispensable. By evaluating their adaptability and accuracy, traders can confidently harness the power of AI for improved decision-making.
Leave a Reply